Can Machines Learn Language Like a Child?
Some concerns about: Grounded Language Acquisition Through the Eyes and Ears of a Single Child
Hello Curious Human!
This week in science — there's a buzz in the air!
The most recent edition of the journal Science featured a new study about an age-old question — how do children learn language?
The results of this research could have significant implications for many areas of research, including psychology, philosophy, and artificial intelligence.
But strong claims need to be backed up by strong evidence. So…
This week in When Life Gives You AI, let’s ask:
Why is there a debate about how children learn language?
What did the researchers do and what did they find that relates to this debate?
What do their findings really tell us about language learning?
Heads up! If you've been eagerly anticipating Part 1 of my series on The Five Most Controversial Ideas in the Study of Consciousness, hang tight! It's all set to land in your inbox next week!
*** Update*** The first author of this Science paper has added a comment to this post. He raises some great points and gives valuable insights that should be read in conjunction with my review.
1. Why is there a debate about how children learn language?
At the core of the mystery about how children learn language is the timeless tussle between nature and nurture. Do our little ones arrive into the world with a built-in knack for language? Or is the world around them—the play, the conversations, the sing-song bedtime stories— enough to shape their learning?
On one side of the debate, we have the nurture crowd who believe that a baby's mind is a blank slate at birth. They’re convinced that language is not preloaded in a baby’s mind — it’s acquired through experience. Through play and conversation, children learn to associate words with their meanings. This learning is set by rewards and punishments. Imagine a baby babbling ‘mama’ for the first time. The parents beam with smiles and cheer with approval. The baby likes this burst of joy, which encourages the baby to repeat the word.
On the other side of the debate, we have the nature crowd, who see each baby coming into the world with a brain that is already pre-wired for language. They argue that there is a problem with the nurture view — children are simply not exposed to enough language to account for the speed at which they learn. Babies must be born with an innate language acquisition device — a universal grammar — that provides them with a natural understanding of language rules.
It is within this arena of intellectual jousting that our Science article attempts to cast some new light.
2. What did the researchers do and what did they find that relates to this debate?
Researchers from New York University set out to understand how babies learn to link words with their meanings. But rather than testing a baby, they turned the spotlight on AI, asking if artificial intelligence, armed with minimal language exposure and few language learning tools, could mirror the language learning feats of a baby.
That is, could an AI learn language like a baby does?
The researchers crafted a clever strategy for their investigation. Instead of training an AI in the usual way — giving it a large banquet of standardised images to train on — they opted to train a model from the vantage point of a child immersed in language learning.
Over a period of 18 months, Baby S wore a helmet with a video camera attached, capturing video footage from the perspective of the child. This amounted to capturing about 1% of Baby S’s waking hours between the ages of 6 to 25 months.
Sifting through the recordings, the researchers found the parts of the recordings that captured moments when the child was spoken to directly — utterances like “you want the ball?”, “You want to pour that in the bucket?” and “It’s a duck and a butterfly”. These snippets amounted to around 61 hours of video footage.
Then the researchers extracted video frames from these segments, which served as the visual input for the model's vision encoder. The audio from these snippets was transcribed into text strings, becoming the language input for the model’s language encoder. In total, this resulted in a hefty dataset of 600,285 video frames and 37,486 text strings.
There were three main steps to this research and two main tests. Let’s break it down.
Step 1
The first step was to do some pre-training for the vision encoder component, using 194 hours of video frames (including the video frames from the 61 hours of video as described above).
The chosen method for the pre-training phase was a self-supervised learning algorithm called DINO (Distillation with NO labels). This clever technique enables the model to learn directly from the data without needing explicit instructions or labels.
It’s important to note that during pre-training, the model was not given any audio or text, it was only given images (video frames).
Step 2
After pre-training, the researchers introduced the model to both the video frames and the text strings, each transcribed from its corresponding video snippet.
Since each text string was associated with multiple video frames, one video frame was randomly selected to be paired with each text string. The model was trained on these pairs, learning to associate text strings (e.g. you want the ball?) with video frames.
Step 3
Following the training phase, it was showtime for the model!
The researcher set up two similar tasks.
In both tasks, the model was given a single word, like ‘ball’. The challenge for the model was to choose the matching image out of four possible options (see Figure 1).
Task 1
In Task 1 (Figure 1C), the four options were images from the original dataset (but not the same images used during training)
Task 2
In Task 2 (Figure 1D), the images were from a different dataset that the model had never seen before.
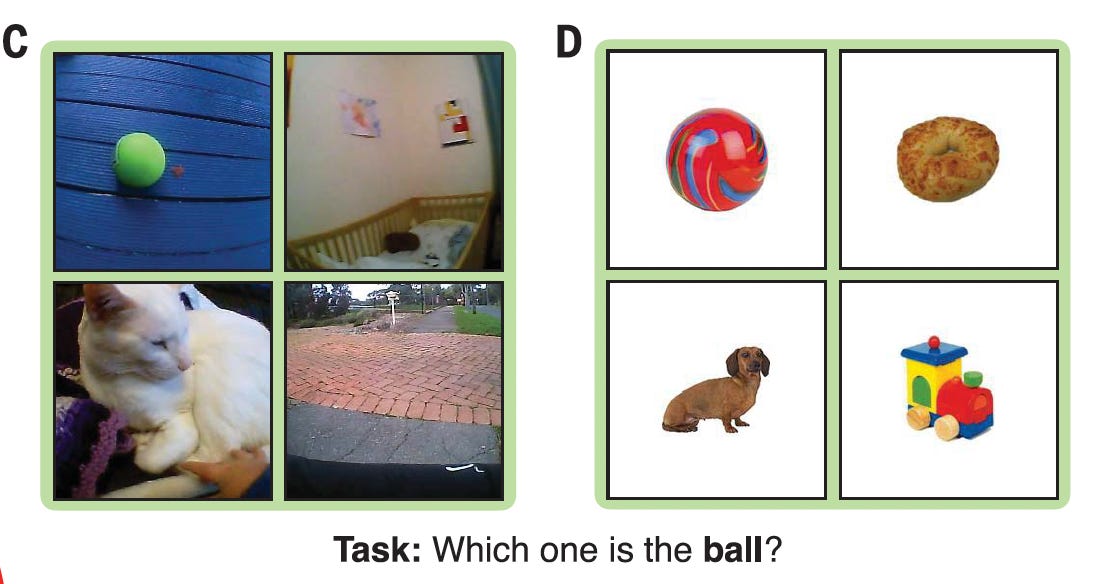
What they found
On Task 1, the model performed the task for 22 different categories (e.g., sand, puzzle, ball, basket, hand, cat, crib, etc.) and was correct 61.6% of the time. Given there were four options — if the model were guessing, we’d expect it to choose the correct answer about 25% of the time. So this performance is not too bad. Indeed, it performed almost as well as a much larger model trained on a lot more data.
The results for Task 2 were not as impressive — but the model still performed above chance. It identified objects across 64 different categories and was correct about 34.7% of the time, which, while lower than its performance on Task 1, still showed some learning.
What the researchers say it means
The researchers suggest their findings are more aligned with the nurture view of language acquisition. While they recognise that the success of their model does not rule out a more sophisticated form of learning, especially forms of learning that show up later in a child’s development, they suggest their findings show that these more sophisticated forms of learning are not essential for a child to learn words through experience.
3. What do their findings really tell us about language learning?
Like most buzzworthy articles, this work is not without its critics. Four main concerns have been raised. The first two relate to the way the researchers interpret their findings. The third and fourth raise issues about the methods that were used.
Concern 1: Did the model learn like a baby does?
In real-life scenarios, a baby is exposed to a continuous stream of sensory input, including visual scenes, sounds, and language. Their input is often cluttered and unstructured and can be overwhelming in its volume and complexity. A critical part of a baby's learning process involves parsing this input, identifying patterns, and determining what information is relevant or important — learning to filter out noise and focus on meaningful signals.
In contrast, the model is trained on data that has already been curated and structured to some extent. The images are frames extracted from videos, and the utterances are transcribed into segmented parts (words) which are presented as text. This pre-processing removes at least one layer of complexity that a baby's cognitive system naturally manages. It means that the model doesn't have to deal with the full extent of variability, noise, and irrelevant information that a baby does.
In other words, a significant portion of the sorting and filtering work that a baby must do to acquire language has been done for the model. Essentially, the model was able to piggyback on an already strong language recognition model — the human researchers.
Concern 2: Did the tests measure language learning?
The researchers were primarily focused on the model’s ability to link words with their meanings. But learning a language is not just about learning words and their associations. It’s also about understanding grammar and knowing how to combine words together according to the rules of the language. There’s a difference between “the butterfly on the block” and “the block on the butterfly”. The words are exactly the same, but the order of those words makes the meaning different. Kids somehow learn this.
Associating words with their respective meanings is a skill children start learning during the early stages of language development. Children don’t usually show signs of understanding grammar until they’re about 2 and a half. The researchers did not set out to test the model’s ability to learn grammar, nor did they make any claims that the model did learn grammar.
But the ability to learn grammar is at the heart of the nature vs nurture debate. The nature crowd believes that we are born with an innate device — a universal grammar — that is essential for language learning. Whether the model can learn anything about grammar is a question for future research.
Concern 3: Is pre-training a blank slate?
Some readers might be wondering whether the pre-training phase might have given the model some structure — something akin to the innate concepts proposed by the nature camp.
The DINO algorithm, used during pre-training, does indeed provide a kind of structure or foundation for the training phase, but only for visual information. This algorithm works by training a model to recognise and emphasise the most important features of an image. It does this by comparing multiple, slightly altered versions of the same image. This method allows the model to teach itself what's important in an image, refining its skills without needing explicit labels or guidance.
The learning during the pre-training phase (Step 1) makes the subsequent training phase (Step 2) more effective because the model isn't starting from scratch—it's able to build on a pre-learned understanding of the data.
The researchers did perform a blank slate test — an alternative model that was trained from scratch — without pre-training. This model performed surprisingly well on Task 1, choosing the correct image 58.3% of the time (chance = 25%). The results from this blank slate test suggest that the pre-training phase was not required for learning.
But a critic might question the results of this blank slate test by raising the next concern.
Concern 4: Was the model overfit?
When doing machine learning, we split the entire dataset into the data we want to use to train our model (training data), and the data we want to use to test our model (testing data). It’s important that we make sure that the training data is different from the testing data. This is because we want a model that can learn to generalise — that is, we want our model to be able to learn new things and then apply that learning to new, unseen situations.
If we use the same data for training the model that we use for testing the model, we don’t really have a way to distinguish between the model memorising the answers and the model learning how to apply that learning.
It's like a teacher giving students a practice exam to prepare for a real exam, and then giving the students the same practice exam as the real exam. A student could perform really well on the real exam, by memorising the questions and answers from the practice exam. The teacher would have no way of knowing which students really understood the underlying concepts and which ones memorised the answers.
When a model performs exceptionally well on a test because it has learned the specific details of the training data instead of the underlying concepts, it is called Overfitting the Model.
In the study, the video frames used during pre-training (Step 1), training (Step 2) and Task 1 of the testing phases (Step 3) were extracted from the same video footage.
The authors do acknowledge that there was overlap between training and testing data but note that they tested the similarity between images and concluded that the similarity was minimal — only 1% of the frames directly overlapped, and approximately 5% indirectly overlapped.
You can see some example frames in Figure 3 (note that for our purposes Evaluation Frame means Test Frame in this Figure). The researchers used a score to evaluate how similar each image was to other images. Images that scored more than 0.95 were considered to be indirectly overlapping. According to this criteria, the column furthest to the left in Figure 3, with a score of 0.99, is indirectly overlapping (or perhaps directly overlapping) and all other pairs were considered not overlapping.
Note: Figure 3 is part of Figure S.8 in the Materials and Methods section, which is free to download.
A critic might respond that even if the researchers were able to use non-overlapping frames between the training and testing phases, the frames from a given scene are not that different.
Imagine a scene from a park where Baby S is asked, “You want to pour that in the bucket?” The utterance, “You want to pour that in the bucket?” is associated with, on average, 16 video frames. Frames 1 through 16 are not independent — they form a sequence with gradual changes. For example, if you were given frame 1 and frame 16, you might be able to estimate the intermediate frames (2 through 15). You could do this based on the content and changes observed between frame 1 and frame 16. There are also a lot of other similarities between frames 1 through 16, like lighting and colour distribution, that could be used to categorise the images. These differences have nothing to do with the underlying concept of ‘bucket’.
A critic might argue that by including similar video frames for training (Step 2) and for Task 1 (Step 3), the results from Task 1 can’t really tell us much about whether or not the model has really understood the underlying concepts.
What we really need to do is look at the results from Task 2. Here, as you will recall, the model was trained on video frames from the Baby S dataset but then tested on a different dataset that the model had never seen before. When the model was asked to perform this more general task, performance dropped to 34.7% — which is still above chance performance (25%) but quite a lot lower than the model’s performance on Task 1 (61.6%).
You might be thinking — well that’s still learning. It’s impressive — yes! Especially given that the model was given such little input.
But if we want to say something about the nature vs nurture debate, we have to be sure we understand what’s going on here.
The model used to perform Task 2 went through pre-training. This, some might argue, is not a blank-slate model. The test we want to run is the blank-slate model (no pre-training) on Task 2. Unfortunately, the study does not report the results of such a test.
The Sum Up
The researchers recognize that, as with all studies, theirs has its limitations. They view their current work as an initial phase and plan to advance their research further. One of their next steps is to include spoken language.
This is an exciting area of research, and I’m looking forward to following their work.
In the next issue…
The Self within Self
We often think of consciousness as a movie playing in our heads — like there’s a little person that sits behind our eyes, watching our experiences on a big screen — maybe even directing the show. But when we look inside, of course, we find no miniature version of ourselves — no little screen.
Where does this feeling come from? Why is it so wrong, and what’s really going on?
In the next issue of When Life Gives You AI, I’ll explore the homunculus fallacy — what is it? Whether it’s all that bad? And what it can tell us about conscious AI?
Find it in your inbox on Tuesday, February 13, 2024.
Thanks Nick! Yes, sometimes these AI models can feel like magic. It's tricky isn't it? Ideally we want to build models that tell us something about the real world. I agree -- AI of this type is an analogy of sorts, but like all good analogies (and bad ones) they can only go so far. The trick is trying to figure out what the models are actually explaining.
Hi Suzi, first author of the Science paper here! I just wanted to say thanks for this fantastic write-up of our work and really diving into some of the details of our training and evaluation set-up. I’m mostly in agreement with the concerns you’ve listed here, but I did want to add a few additional comments and provide some additional context for some of our choices to your readers:
- You mention a language acquisition device, or a universal grammar, as an example of the nature view. Since our work focuses mostly on the problem of lexical acquisition rather than grammar, there are other beliefs more in line with the nature view that are worth mentioning as well, such as knowing the set of visual referents that a concept could refer to at birth (e.g. an innate view of concepts), or having strong constraints about how to extend or generalize a word (e.g. based on its shape, or only considering whole objects instead of parts), or assuming that each word has a different meaning (the principle of contrast). We do not explicitly include any of these mechanisms into our model, its primary method of learning these mappings is purely by the co-occurrences between visual and linguistic information.
- Concern 1: I agree that we do remove some layers of complexity compared to the raw multi-sensory stream of what a baby receives, by only considering the paired moments between spoken utterances and visual input, as well as passing in the transcribed utterances, but I would argue that many of the other well-known models that purport to study cross-situational word learning make even more assumptions, such as removing the visual aspect of the problem entirely! See https://www.sciencedirect.com/science/article/pii/S0010028512000795?casa_token=UOzXoHdHMEMAAAAA:1jPkoacJooE6UIB7uDU_ah8GPBnCZYLbDqKMLHhA5p37gJ-ZQ-x2JVZAnZEThagP9Sj2UlU or https://journals.sagepub.com/doi/full/10.1111/j.1467-9280.2009.02335.x?casa_token=wT08hOSACawAAAAA%3APrxJEByZR7clWbKTHhZPXlu7aG-aBXl_4ZdraIOZ039pzYL8bGlE-Ss7Silk22-tLzalF4nUJGBN. Additionally, this kind of pre-processing is also common in multimodal machine learning. It is quite common to have some amount of pre-processed or annotated data to also feed into the model (see examples from the Ego4D challenge that also uses an egocentric video dataset: https://ego4d-data.org/docs/challenge/). Nevertheless, even with some of these pre-processing steps, our work still provides the largest and most representative subset of one child’s experience to date, and I think demonstrating that learning is possible from this is still a huge advance for the field.
- Concern 2: We only study the problem of learning word-referent mappings because this problem is so big it has its own subfield within cognitive science! Of course, we recognize that grammar and the compositionality of language are also very important and acquired by children later on, but we believe that it made sense to start at the very beginning. Nevertheless, my co-author Wentao Wang led a separate project looking at learning these other aspects of language from the same dataset which you can find here: https://onlinelibrary.wiley.com/doi/abs/10.1111/cogs.13305, demonstrating that some aspects of syntax and semantics can also be learned from one child’s linguistic input. Therefore, I think it would be relatively straight-forward to train a model that learns both word-referent mappings and grammar from this single dataset. Although we have not explicitly pursued this just yet, perhaps we might need to do so given how many people have raised this issue as a potential gotcha! Separately, your example of differentiating between “the butterfly on the block” vs. “the block on the butterfly” is actually very challenging! The WinoGround paper (https://openaccess.thecvf.com/content/CVPR2022/html/Thrush_Winoground_Probing_Vision_and_Language_Models_for_Visio-Linguistic_Compositionality_CVPR_2022_paper.html), looks at this problem in great detail, and finds that even state-of-the-art multimodal models trained on millions of datapoints still fail to solve this problem.
- Concern 3: This concern might be a bit of a conceptual difference. To me, I think of the blank state as Step 0: when the model’s weights are randomly initialized, but I treat both Steps 1 and 2 (as you’ve laid them out) as separate pre-training stages (rather than pre-training and training), since children do receive and learn from visual experience in the first few months of life prior to acquiring any language (Step 1), but then that gets refined with the addition of language input (Step 2). So to me, I don’t think that the visual representations of the model after Step 1 necessarily constitute innate concepts, since those representations were still learned from data. It would only be innate if those concepts were there at Step 0 (in the random weights of the neural network prior to receiving or learning from any data, either vision or language).
- Concern 4: These are all really good points regarding overlap! Note that in addition to a different sampling process to extract the evaluate frames (that were done independently of the spoken language, only via the visual content), the pre-training task in Step 2 (matching video frames to entire utterances) is also slightly different to the evaluation task (matching single words to video frames), nor was this evaluation dataset used during any of the pre-training stages, so it is not the standard kind of overfitting you see in machine learning. However, as our overlap analysis shows, the fact the frames are sampled from the same set of videos may have raised the performance of the model slightly, but it doesn’t take away the fact that the model does generalize to images outside of its training distribution. I think it's this finding which gives me strong confidence that it is learning a generalizable mapping between words and images, and not merely overfitting in the traditional sense (which would fail to display this kind of generalization). I agree that its performance is a bit lower in the out-of-distribution evaluation, but that’s because we wanted to include as many possible words to evaluate on (even those the model may have only seen a handful of times!), which did end up lowering the overall average performance. Even then, performance for half of the concepts are above-chance (32 out of 64), which I think is quite promising! One last point: we’re working on training a version of CVCL using an independent set of videos from baby S distinct from the evaluation frames, thus removing the potential overlap issue, so stay tuned!
I hope some of my responses clarify some of the concerns you’ve raised about our work, and happy to answer any other questions you might have!